The Future of IT: Integrating Malware Machine Learning for Enhanced Security
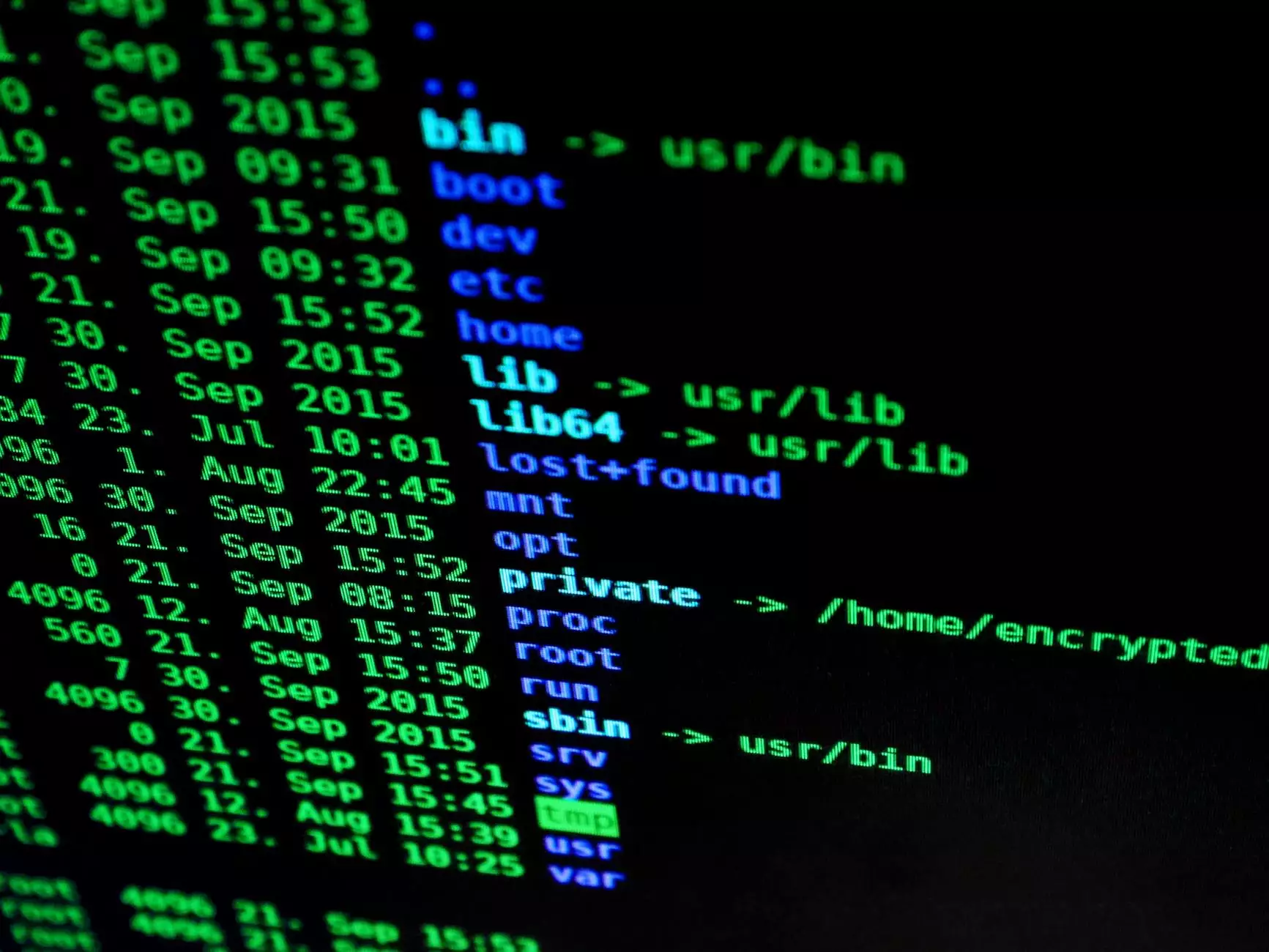
In today's digital age, cybersecurity is more critical than ever before. As businesses increasingly rely on technology, the threat landscape continues to evolve, presenting new and sophisticated challenges. Among these challenges, the integration of malware machine learning stands at the forefront of modern IT services and security systems. This innovative approach not only enhances the detection of threats but also ensures a resilient infrastructure that can withstand attacks.
Understanding Malware and Its Impact on Businesses
Malware, short for malicious software, is designed to disrupt, damage, or gain unauthorized access to computer systems. The impact of malware on businesses can be devastating, leading to significant financial losses, data breaches, and damage to reputation. Traditional methods of cybersecurity often fall short as they rely on known signatures, leaving organizations vulnerable to new and unknown threats.
The Evolution of Malware Threats
Over the years, malware has evolved from simple viruses to complex forms of attack, including ransomware, trojans, and spyware. Each new generation of malware is designed to evade detection by conventional security measures, making it increasingly challenging for businesses to protect their assets. This is where malware machine learning comes into play.
What is Malware Machine Learning?
Malware machine learning refers to the application of artificial intelligence (AI) techniques to improve the detection and prevention of malware attacks. By analyzing vast amounts of data, machine learning algorithms can identify patterns indicative of malicious behavior without relying solely on known signatures.
How Does It Work?
The process begins by gathering data from various sources, including:
- User activity logs
- Network traffic
- File attributes
- Historical malware samples
Machine learning models are then trained on this data to recognize normal behavior and detect anomalies. When potential threats emerge, the system can react accordingly, blocking potential infections before they can cause harm.
Benefits of Implementing Malware Machine Learning in Your Business
The integration of malware machine learning into your IT services offers numerous advantages:
1. Enhanced Detection Rates
One of the most significant benefits is the improved detection rates of malware. Traditional antivirus solutions often struggle with zero-day vulnerabilities and sophisticated attacks. Machine learning can adapt and learn from new threats, leading to higher accuracy in identifying malware.
2. Reduced False Positives
False positives can hinder productivity by incorrectly flagging legitimate software as risks. Malware machine learning dramatically reduces these instances, allowing IT teams to focus on genuine threats rather than sifting through unnecessary alerts.
3. Proactive Threat Prevention
With real-time threat detection, businesses can adopt a more proactive stance to cybersecurity. Instead of merely responding to attacks, they can anticipate and block potential threats effectively, reducing the chances of a successful breach.
4. Scalability
As organizations grow, so do the complexities of their IT environments. Machine learning models can scale with the organization, adapting to new data and evolving threat landscapes without needing comprehensive manual updates.
Challenges of Malware Machine Learning
While the benefits are considerable, implementing malware machine learning also presents challenges that businesses must navigate:
1. Data Privacy Concerns
Collecting vast amounts of data raises significant privacy concerns. Organizations must ensure they comply with regulations such as GDPR when handling sensitive data.
2. Complexity of Implementation
Establishing a machine learning system requires considerable expertise, both in technical skills and in the understanding of machine learning principles. Companies must invest in training or hiring skilled professionals to manage these systems effectively.
3. Evolving Threats
As malware creators become more sophisticated in their tactics, machine learning models need constant updates and retraining to stay ahead of emerging threats. This ongoing commitment can be resource-intensive.
Integrating Malware Machine Learning into Your Security Strategy
To fully leverage the power of malware machine learning, organizations must integrate it into their broader security strategy. Here are key steps to consider:
1. Assess Current Security Posture
Understanding your existing security measures and their effectiveness is crucial. Conduct a thorough assessment to identify weaknesses and gaps that machine learning could address.
2. Choose the Right Tools
Numerous tools and platforms are available that utilize machine learning for threat detection. Research and select the solution that best fits your organization’s specific needs and infrastructure.
3. Invest in Training
Ensure your IT team is proficient in using machine learning tools. Regular training and workshops will help them stay updated on the latest trends and techniques in cybersecurity.
4. Continuous Monitoring and Evaluation
Ongoing monitoring is essential to maintain the effectiveness of machine learning models. Regularly evaluate their performance and adjust them based on new data and emerging threats.
Case Studies: Success Stories of Malware Machine Learning
Many companies have successfully integrated malware machine learning into their cybersecurity strategies. Here are some notable examples:
1. Company A: Reducing Incident Response Times
A large financial institution implemented a machine learning-based solution that reduced their incident response times by 60%. By quickly identifying and isolating threats, they minimized the potential impact on their operations.
2. Company B: Enhancing Threat Detection
A tech company saw a 75% increase in threat detection rates after integrating machine learning into their security systems. This allowed them to act swiftly, protecting sensitive customer data and maintaining trust.
The Future of Malware Machine Learning
The future of malware machine learning is promising, as technology continues to advance at a rapid pace. Organizations must remain adaptable, leveraging the latest developments in AI and machine learning to stay one step ahead of cybercriminals.
1. Advancements in AI
As AI technology matures, we can expect significant improvements in the sophistication of malware detection algorithms. This will lead to even more accurate predictions of potential threats.
2. Collaborative Defense Mechanisms
Businesses will increasingly share threat intelligence, utilizing machine learning to analyze data from multiple sources. This collaborative approach will enhance collective cybersecurity defenses.
Conclusion
In conclusion, integrating malware machine learning into your IT services is not just a trend; it's a necessary evolution in the fight against cyber threats. By adopting this advanced approach, businesses can significantly improve their security posture, reduce the risks of malware infections, and protect their valuable assets. Investing in machine learning solutions for threat detection should be a priority for any organization aiming to thrive in the digital landscape. As threats continue to evolve, so must our strategies, and machine learning offers a robust foundation for future cybersecurity efforts.