Unlocking the Power of Image Datasets for Classification
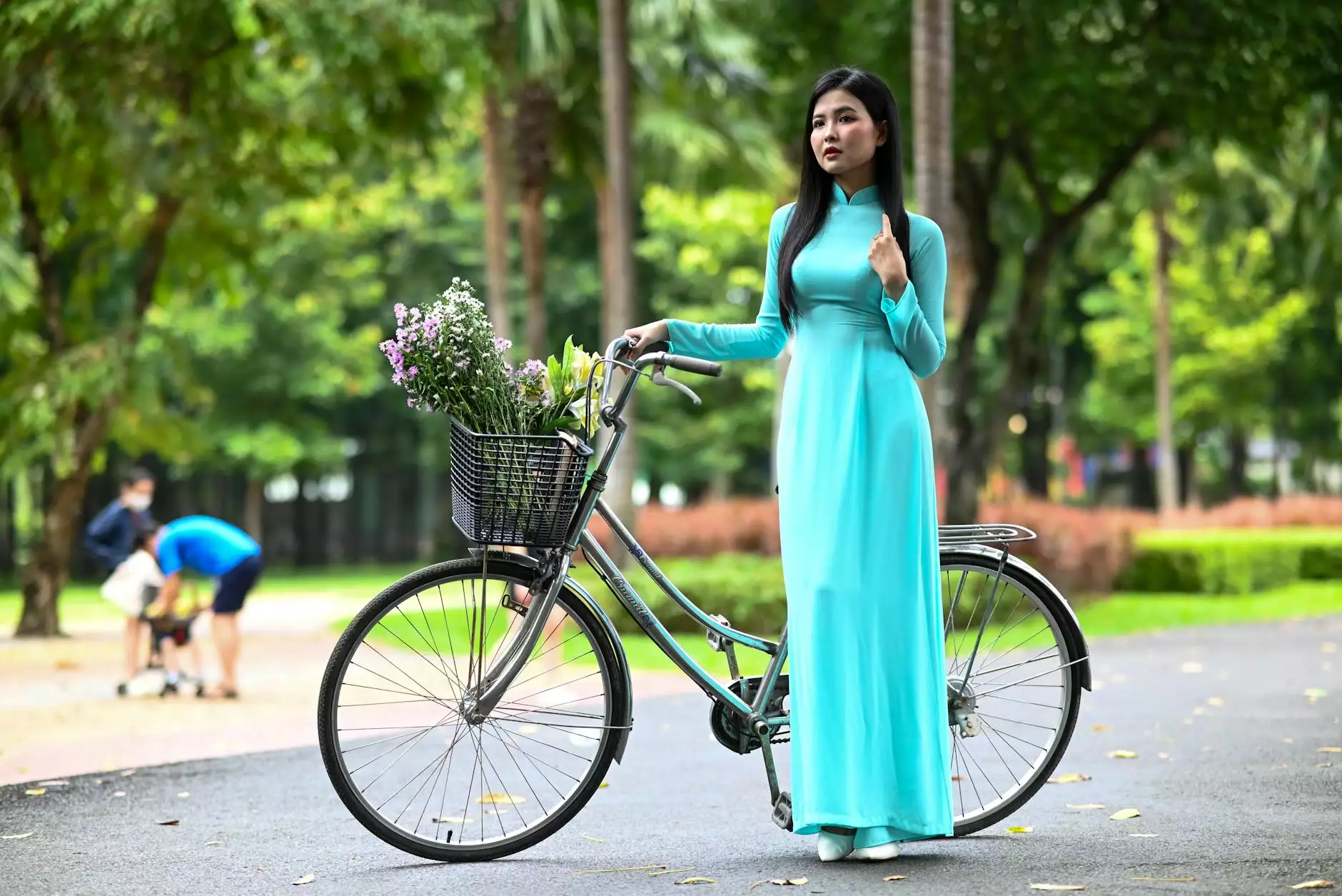
The digital era has ushered in a revolution in the way businesses operate, utilize data, and make informed decisions. One critical component in this vast data landscape is the concept of image datasets for classification. These datasets not only enhance machine learning models but also enable businesses to derive actionable insights that can lead to growth and innovation.
Understanding Image Datasets for Classification
At its core, an image dataset for classification is a collection of images intended to be used for training algorithms to recognize and categorize visual data. The classification is typically based on pre-defined labels, enabling the model to learn patterns and features associated with specific categories.
Why Are Image Datasets Important?
- Enhancing Machine Learning Models: With high-quality image datasets, machine learning models can achieve greater accuracy and efficiency.
- Facilitating Automated Processes: Businesses can automate image recognition tasks, reducing manual work and increasing productivity.
- Driving Better Decision Making: By interpreting visual data more effectively, organizations can make more informed decisions.
The Role of Data Annotation in Building Effective Image Datasets
Data annotation is a pivotal aspect of creating image datasets for classification. It involves labeling images to provide the necessary information required for training algorithms. The accuracy of the annotation directly impacts the performance of the resulting machine learning model.
Types of Data Annotation Techniques
There are various methods employed for data annotation, each suited to different types of classification tasks. Some of the most common techniques include:
- Bounding Boxes: Used to define a specific area in an image, often utilized in object detection tasks.
- Polygon Annotation: For intricate shapes or objects, polygon annotation offers detailed outlining that increases accuracy.
- Semantic Segmentation: This technique involves labeling each pixel in an image, providing granular classification for complex datasets.
- Landmark Annotation: Involves marking specific points of interest on an object, widely used in facial recognition tasks.
Choosing the Right Data Annotation Tool
Selecting an appropriate data annotation tool is crucial in creating robust image datasets. At KeyLabs AI, we provide cutting-edge data annotation platforms designed to streamline the annotation process, ensuring high-quality results. Our tools are equipped with features that enhance productivity and accuracy:
- User-Friendly Interface: Simplified interfaces that enable teams to start annotating effectively within minutes.
- Collaboration Features: Facilitate teamwork through real-time collaboration, allowing multiple users to work on the same project seamlessly.
- Quality Control Mechanisms: Built-in validation tools to guarantee the accuracy and consistency of annotated data.
Real-World Applications of Image Datasets for Classification
The applications of image datasets for classification span a multitude of industries, showcasing their versatility and importance:
1. Healthcare
In the healthcare sector, image classification plays a significant role in diagnostics. Medical imaging datasets help train AI models to identify diseases through X-rays, MRIs, and other imaging modalities. By accurately classifying images, healthcare practitioners can enhance their diagnostic capabilities, improving patient outcomes.
2. Retail and E-commerce
Retailers leverage image classification to categorize products automatically. By employing machine learning models to recognize products in images, businesses can streamline inventory management, enhance search functions, and improve customer experiences through personalized recommendations.
3. Automotive Industry
Autonomous vehicles rely heavily on image datasets for classification. These vehicles must recognize various elements in their environment, such as pedestrians, road signs, and other vehicles. Comprehensive image datasets are essential for training models that ensure safety and efficiency on the roads.
Building High-Quality Image Datasets
Creating an effective image dataset involves meticulous planning and execution. Here are several key steps to consider:
- Define the Objective: Clearly outline the purpose of the dataset and the classification tasks intended.
- Gather Diverse Data: Collect images from a variety of sources to ensure the dataset covers all aspects needed for robust classification.
- Implement Thorough Annotation: Use expert annotators or reliable annotation tools to achieve high-quality labeling.
- Regularly Update the Dataset: Continually refine and expand the dataset to adapt to new challenges and ensure ongoing effectiveness.
KeyLabs AI: Your Partner in Data Annotation
At KeyLabs AI, we understand the importance of image datasets for classification and offer a comprehensive data annotation platform that caters to various industries. Our platform is designed to meet the needs of businesses looking to harness the power of data in the age of AI.
Why Choose KeyLabs AI?
KeyLabs AI stands out for several reasons:
- Expertise: Our team possesses extensive experience in data annotation and machine learning, ensuring that your projects are managed by knowledgeable professionals.
- Scalability: Whether you are a startup or a large enterprise, we offer scalable solutions tailored to your specific needs and project sizes.
- Cost-Effectiveness: We provide competitive pricing without compromising on the quality of our services.
- Customization: Our tools and services can be tailored to suit the unique requirements of different projects, ensuring optimal results.
The Future of Image Datasets for Classification
As technology continues to evolve, the future of image datasets for classification looks promising. With advancements in computer vision and machine learning, we can expect:
- Increased Automation: More businesses will harness automated systems for data annotation, enhancing speed and efficiency.
- Greater Use of Synthetic Data: The production of synthetic images to augment real-world datasets will become more prevalent, addressing data scarcity issues.
- Enhanced AI Models: Future models will likely leverage improved algorithms, resulting in higher accuracy and broader application ranges.
Conclusion
The significance of image datasets for classification cannot be overstated. They are fundamental in the training of efficient machine learning models, aiding businesses across multiple domains to succeed and innovate. By utilizing advanced data annotation tools and platforms like those offered by KeyLabs AI, companies can effectively harness the power of their visual data, leading to improved performance and outcomes.
Investing in high-quality image datasets and reliable annotation processes is vital for any organization aiming to stay competitive in today's data-driven landscape. As the field of AI continues to progress, now is the time to unlock the potential that lies within your image data.