The Comprehensive Guide to the Data Annotation Process
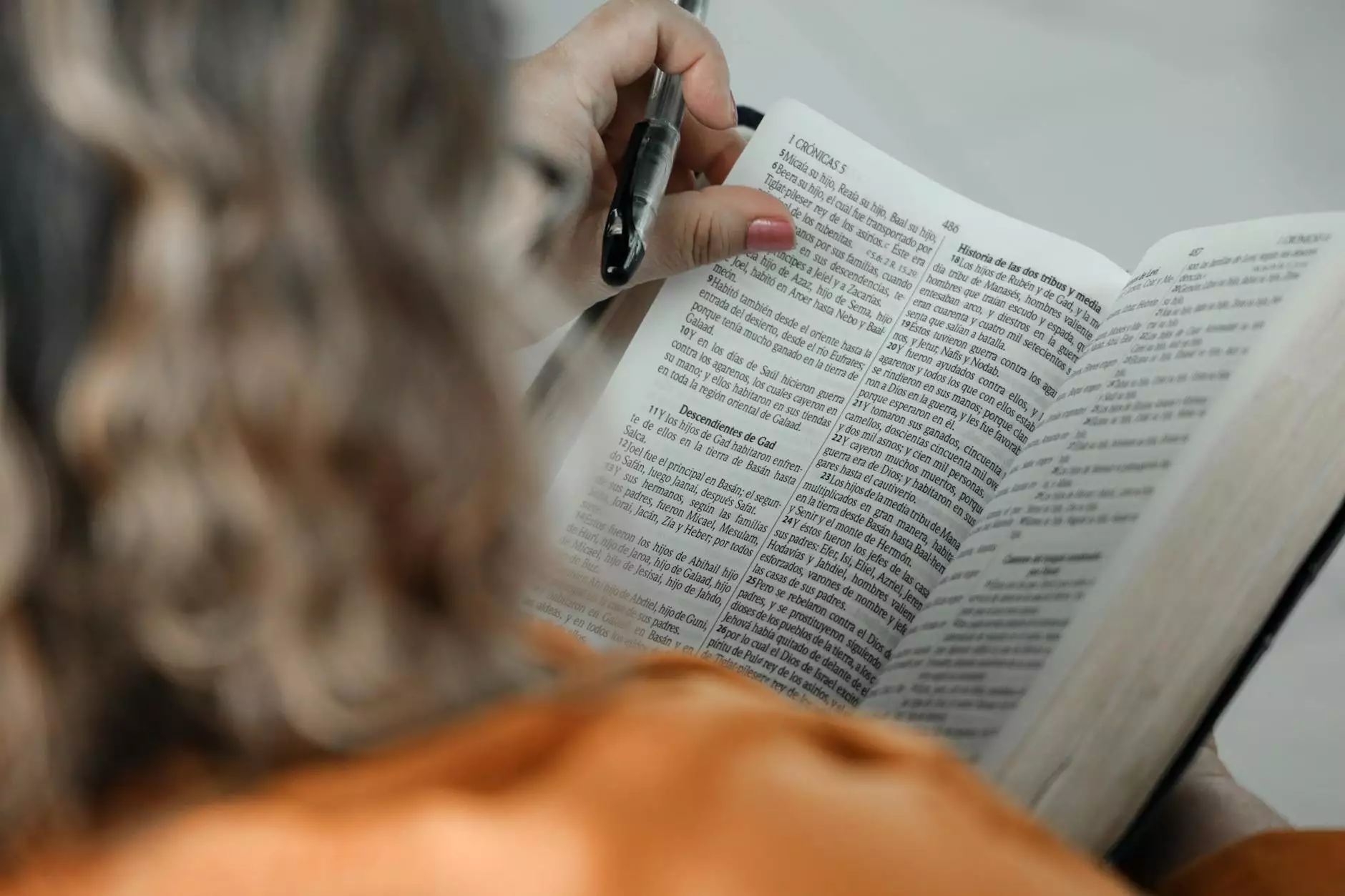
The data annotation process is a critical component in the field of artificial intelligence and machine learning. As businesses increasingly rely on data-driven technologies, understanding this process has never been more vital. In this article, we will delve into the intricacies of the data annotation process, its significance, the types of annotation, and how it enhances software development, especially within the realm of Keymakr.
Understanding Data Annotation
Data annotation involves labeling data to make it more meaningful and usable for algorithms. This is foundational for systems that utilize machine learning, as algorithms learn from the annotated data to make predictions or decisions without human intervention.
Importance of Data Annotation
Data annotation serves multiple crucial purposes in modern software and technology solutions:
- Enhancing Model Accuracy: Properly annotated datasets improve the accuracy of machine learning models, enabling them to learn effectively from the data.
- Training AI Systems: For AI systems, the quality of training data directly correlates with performance; well-annotated data ensures that these systems operate efficiently.
- Scalability: As businesses scale their operations, the need for robust, annotated datasets becomes paramount to maintain accuracy across diverse scenarios.
Types of Data Annotation
The data annotation process can take various forms, each suited to different types of data and applications. Here are some of the most common types:
1. Image Annotation
Image annotation involves labeling objects or features within images. This is particularly important for computer vision tasks. There are several forms of image annotation:
- Bounding Boxes: Drawing rectangles around objects to identify their location.
- Semantic Segmentation: Classifying each pixel in the image to delineate classes of objects more effectively.
- Keypoint Annotation: Identifying specific points on an object, such as joints on a human body, to enable pose estimation.
2. Text Annotation
Text annotation involves labeling parts of a text document to enhance natural language processing applications. This can include:
- Named Entity Recognition (NER): Identifying proper nouns and classifying them into categories such as people, organizations, and locations.
- Sentiment Analysis: Annotating texts to determine the sentiment expressed within the content.
- Part-of-Speech Tagging: Labeling words with their corresponding parts of speech (e.g., nouns, verbs, adjectives).
3. Audio Annotation
In audio annotation, sounds or speech are labeled for various purposes, including:
- Speech Recognition: Creating datasets for voice recognition systems by identifying and labeling spoken words.
- Sound Event Detection: Labeling sounds to enable AI models to recognize specific events in audio recordings.
The Data Annotation Process: Step-by-Step
Now that we've covered the types of annotation, let’s break down the data annotation process into clear steps to understand how it is executed effectively:
Step 1: Data Collection
The first stage involves gathering relevant data. This data can come from various sources, including:
- Surveys and questionnaires
- Publicly available datasets
- Self-generated datasets from user interactions
Step 2: Data Preprocessing
Once data is collected, it often requires preprocessing. This includes cleaning the data (removing duplicates, correcting errors) and organizing it into a manageable format. Preprocessing is crucial because it ensures that only high-quality data enters the annotation phase, significantly enhancing the outcome.
Step 3: Annotation Task Assignment
Next, organizations typically assign specific annotation tasks to skilled annotators or use automated tools. Choosing the right approach depends on:
- The type of data being labeled
- The required accuracy level
- Available resources, both human and technological
Step 4: Annotation
During the annotation step, data is labeled according to the chosen method. This can be done manually or through automated systems, depending on the scale and complexity of the task. Manual annotation often yields higher accuracy, while automated systems can process large volumes of data more quickly.
Step 5: Quality Assurance
After the initial annotation, quality assurance is essential to ensure that the labels are accurate and consistent. This may involve:
- Reviewing annotated data by additional annotators
- Implementing statistical checks on the consistency of labels
- Iterating on the annotations based on quality feedback
Step 6: Integration
Once the annotated data passes quality checks, it can be integrated back into the larger dataset. This can then be utilized for training machine learning models, refining AI algorithms, or serving specific software solutions.
The Benefits of Efficient Data Annotation
Implementing an efficient data annotation process can provide numerous benefits to businesses, particularly in software development. Here’s how:
- Increased Efficiency: Streamlined data annotation processes lead to quicker turnaround times for project completion.
- Enhanced Competitive Edge: Organizations that master the data annotation process can innovate faster than their competitors.
- Better Decision Making: Well-annotated data offers deeper insights, allowing for better business decisions and strategies.
- Cost Savings: Reducing errors during the annotation process leads to lower costs associated with model retraining and troubleshooting.
Challenges in the Data Annotation Process
While the data annotation process has many advantages, there are also significant challenges that organizations must navigate:
1. High Labor Costs
Manual annotation of large datasets can be labor-intensive and expensive. This often leads businesses to seek a balance between quality and cost, which can be difficult to achieve.
2. Ensuring Consistency
When multiple annotators are involved, maintaining consistent labeling can be a challenge. Techniques such as comprehensive training and clear guidelines are critical to address this issue.
3. Dealing with Ambiguous Data
Some data may be inherently ambiguous or subjective, leading to differing interpretations. Ensuring that annotators have a comprehensive understanding of the context is vital.
Future Trends in Data Annotation
The future of the data annotation process is exciting, with several trends poised to shape its evolution:
1. Automation and AI Integration
The integration of automated tools powered by artificial intelligence is likely to significantly enhance the efficiency of the data annotation process. These tools will continue to develop, providing higher accuracy and reducing the need for manual input.
2. Increased Demand for Specialized Annotation
As industries become more specialized, the demand for targeted annotation services will rise. Businesses will increasingly seek annotators with expertise in specific domains.
3. Crowdsourcing and Community Engagement
Crowdsourcing annotation tasks can potentially lower costs while also engaging a community of contributors. Platforms that facilitate this will become increasingly popular.
Conclusion
The data annotation process is foundational to the development of robust and reliable AI systems. As organizations, particularly in software development like Keymakr, leverage the power of data, understanding and implementing an effective data annotation strategy becomes a critical business asset. By mastering this process, businesses not only enhance their AI models but also ensure that they remain competitive in an increasingly data-driven world.
Investing in high-quality data annotation is not merely an operational expense; it is an investment in the future of AI-driven applications. As technology continues to advance, the importance of accurate data annotation will only grow, making it an essential component of any successful AI and software strategy.